The discussion about common standards in the real estate industry has been going on for decades. Now, Dig-IT Lab is launching its Testbed Xn — an infrastructure designed to speed up the industrialisation of digital features. In this new testbed, Dig-IT Lab and its partners can compare different buildings and digital models, collaborate on standards, and develop sustainable services for smart buildings and cities.
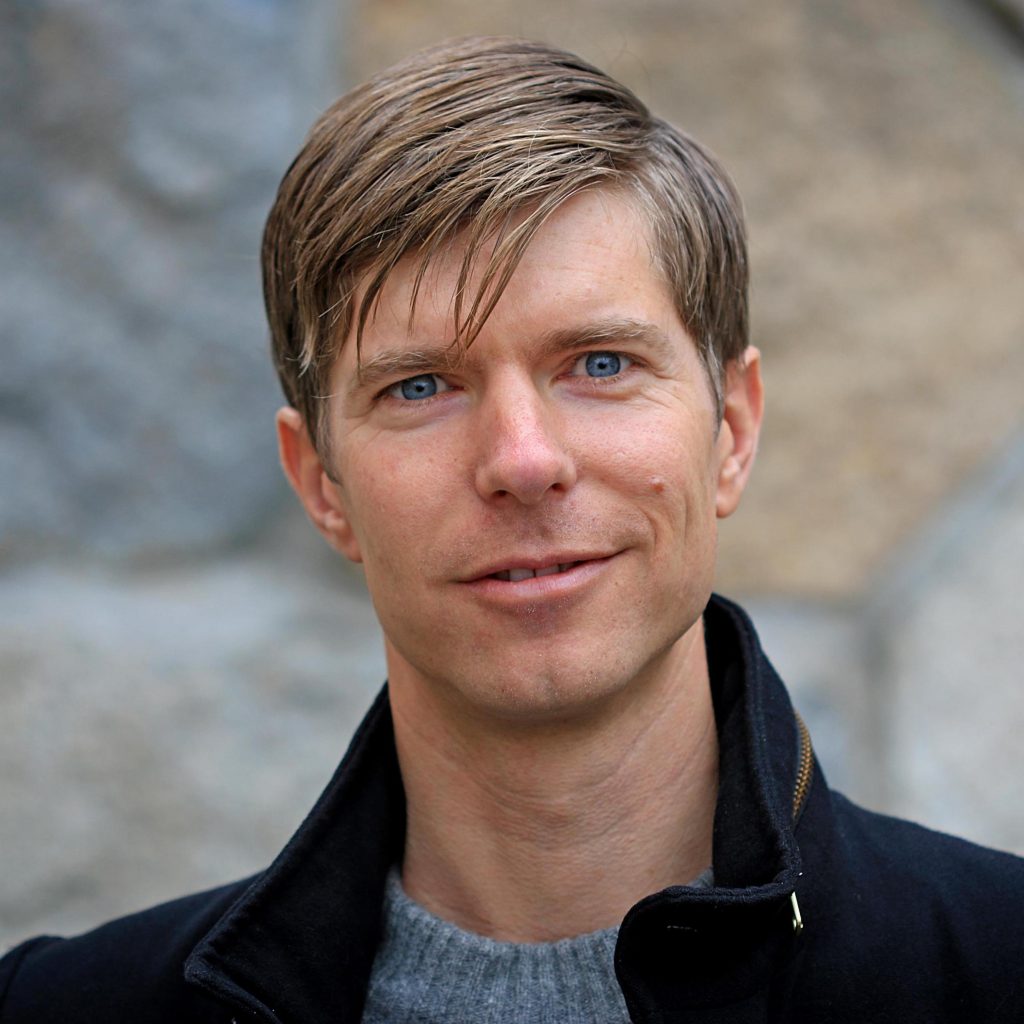
Jonas Anund Vogel, Director at Dig-IT Lab, explains what the new testbed is, who can benefit from it, and why it is so useful to be able to compare buildings.
What does Testbed Xn stand for? And how is it different from Dig-IT Lab’s other testbeds?
We have several centre partners, and they all have their own digital models or ”twins.” We call those Testbed X1, Testbed X2, and so on. Now we want to figure out how we can use all these different testbeds together within Dig-IT Lab, and also build a new shared infrastructure for research and development. So, we are gathering all the Testbed ”X” projects under one umbrella and calling it Testbed Xn. We are aiming to create an environment where we can test and develop functions that could lead to new services for the entire industry. Right now, we can do case studies in individual buildings, but scaling it up is a whole different challenge — and we believe that will take a bigger ecosystem. That ecosystem is Testbed Xn.
Is there anything similar out there today?
As far as I know, there is no digital testbed that focuses on the areas Dig-IT Lab is working on. Testbed Xn can be used for things like AI development, benchmarking, and fault detection, using both “white box models” and “black box models.” It will also have sandboxing capabilities, meaning projects can test everything from disaggregating data — like figuring out energy use for ventilation, heating, and hot water just from the total energy use — to accessing different systems through different data layers. Right now, we have access to data layers from Schneider Electric, Siemens, and ProptechOS, but it can eventually grow into the industry’s own kind of “App Store.”
What are the challenges with creating common standards in the industry?
The conversation about a shared language and naming system in the building sector has been going on for decades. But it is not enough to just name things the same way — you also need an organisation and a business model that can create value from that shared information. Today, different actors have developed their own languages — or “ontologies” — and standards to store and share building data. But pulling out that data and creating real value from it is still hard. Around 96 percent of all data gets thrown away today, and most of the remaining data is unstructured and not very useful for value-creating applications.
Can the testbed help with standardisation?
Yes, we are initiating research and development projects that can help advance the use of common structures and develop new business models. We are also focused on demonstrating the actual benefits of using shared approaches.
You mentioned ontologies — how do they fit into all this?
There are quite a few ontologies out there — like ”Real Estate Core,” ”Brick,” ”Saref,” and ”Haystack.” They are used for different purposes and go into different levels of detail. With Testbed Xn, we want to look at how these ontologies connect to each other and figure out where there are gaps — where there is no language yet. We also want to find out how detailed you need to be in describing different parts to create good enough services. It is really a balancing act between functionality and effort.
Why is it so important to be able to compare buildings?
Being able to compare buildings is important for things like fault detection. If you see that one building uses way more energy than a similar one, that is a red flag — something is wrong, or at least different. Maybe the building is normally only used during the day, 8 to 5, but sometimes there are evening events like parent meetings at a school, or exams on weekends, and then you need ventilation, lighting, and alarms to work properly.
Benchmarking is another interesting use. You can test different functions in digital building models and figure out which service or app works best for that specific building. Other examples of the benefits of common structures include using temperature sensors for fire alarms, telling the cleaning company when rooms need cleaning, and giving tenants heads-up about upcoming renovations. The opportunities are basically endless, and only your imagination is the limit. If we establish a solid foundation for common structures, others can start building services on top of it.
Who will be able to use the testbed — and when?
Anyone who wants to do research and development can use what we are building. However, just like with our physical testbed, KTH Live-In Lab, you need to have a clear purpose and a plan. An application form to apply for access will be available soon, but until then, you can simply reach out to me or one of the deputy directors.
Facts Testbed Xn
Why the ”n”?
In the name, “n” is like the exponent in the mathematical expression ”X^n,” meaning X multiplied by itself n times.
How are digital twins used in the building and real estate industries?
In short, a digital twin is a digital representation of the physical world – in our case, buildings — and it is loaded with real operational data. If the temperature rises in the real world, you will see the same change in the twin. A strength is that you can simulate alternative scenarios in the digital environment.
There is a lot of interest in digital twins in real estate right now, but we are still learning how to unlock their full potential. Going forward, they will play a bigger role throughout the entire building process and help increase property values.
What are “ontologies”?
Ontologies are used to describe and name the different parts, activities, and relationships that matter for buildings — and eventually for smart cities. Say you want a building to be controlled by some third-party function — like an AI engine that optimizes heating and ventilation based on energy prices, user preferences, and maybe even energy storage within an energy community — then you need a common language for all that, in other words, an ontology.
Read more about ontologies in our Publications section: www.digitlab.kth.se/dig-it-lab-publications/